We invite student posters for presentation at the Lucy Family Institute for Data and Society Fall Symposium Wednesday, Oct 27.
See Call for Posters to enter and for more information.
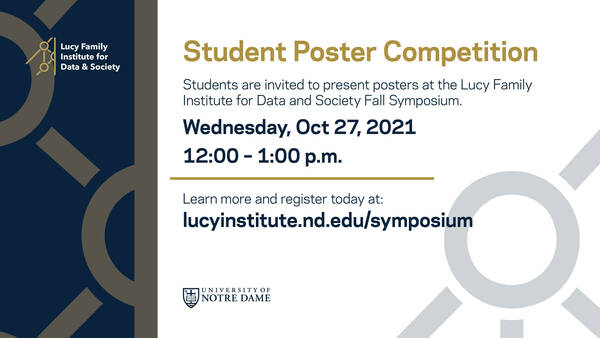
Posters that feature project concepts, new or continuing research in data science, AI, data engineering, computing, applications, and methods that amplify societal expertise in areas of human development, peace accord, ethics, global development, health disparities, and poverty are particularly welcome.
- Oct 20 Student Registration & Poster Entry Deadline EXTENDED!
- Oct 21 3 PM Poster Print Job Submission Deadline
- Oct 22 Student Poster Competition Entries Announced & Abstracts posted
- Oct 27 Lucy Family Institute Fall Symposium