Environment & Climate
The network structure and evolution over time provide interesting insights into the behavior of the Earth’s environmental and climatic systems. For example, ocean climate indicators extracted from the networks have proven to be good predictors of climate variables over land. Currently, we are studying the dynamic behavior and stability of the network over time.
Click the title of the project to view the description.
Patterns of Ship-Borne Species Spread
The spread of non-indigenous species (NIS) through the global shipping network (GSN) has enormous ecological and economic cost throughout the world. Previous attempts at quantifying NIS invasions have mostly taken “bottom-up” approaches that eventually require the use of multiple simplifying assumptions due to insufficiency and/or uncertainty of available data. By instead modeling implicit species exchanges via a graph abstraction that we refer to as the Species Flow Network (SFN), we pursue a different approach that exploits the power of network science methods in extracting knowledge from largely incomplete data.
Education
Predicting STEM Student Retention
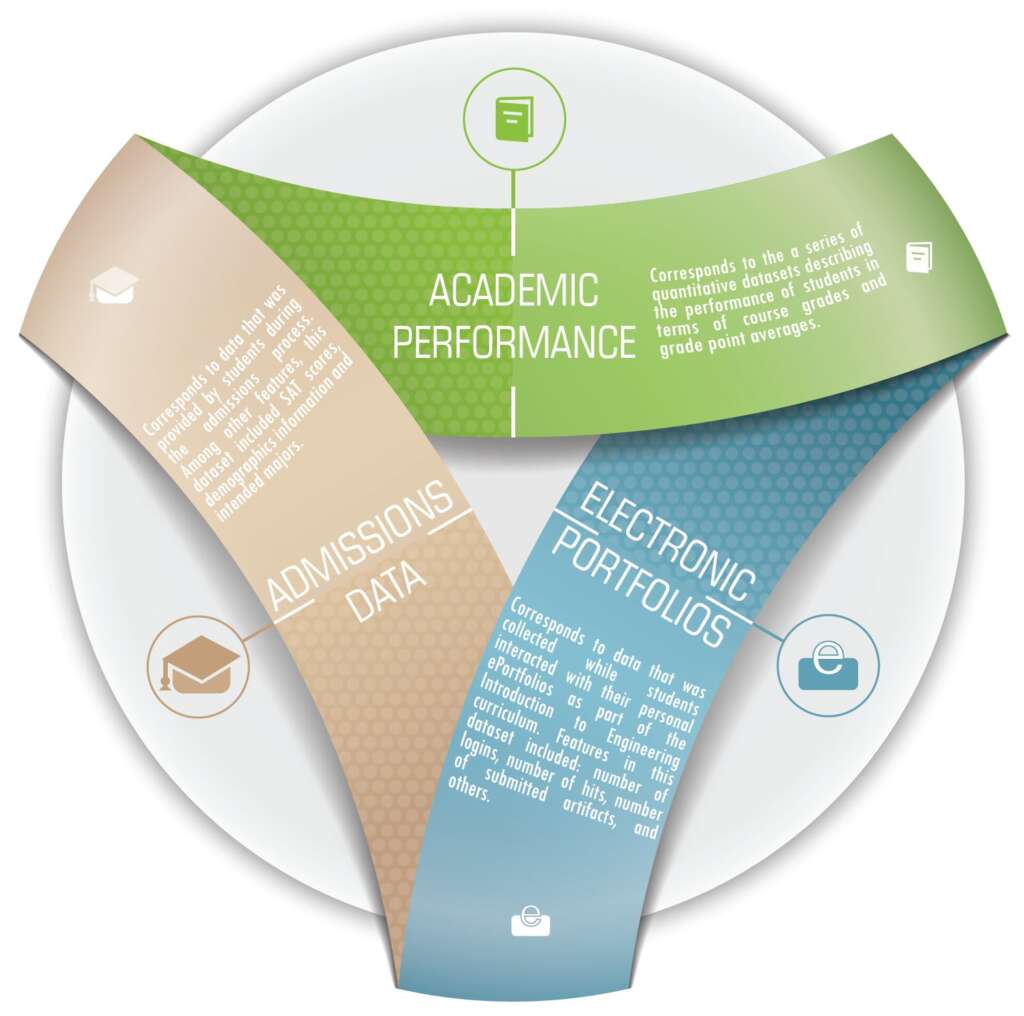
As providers of higher education begin to harness the power of big data analytics, one very fitting application for these new techniques is the prediction of student attrition. The ability to pinpoint students who might soon decide to drop out of a given academic program allows those in charge to not only understand the causes for this undesired outcome but also provides room for the development of early intervention systems. While making such inferences based on academic performance data alone is certainly possible, we claim that in many cases there is no substantial correlation between how well a student performs and his or her decision to withdraw. To address this issue, we aim to derive measurements of engagement from students’ electronic portfolios and use these features to augment the predictions of student attrition.
Identifying Students of Concern in The First Year of Studies Course
The First Year of Studies (FYS) is a required course offered in the flipped classroom format that undergraduate students entering the university need to take and pass in the first year. Thus, it is important to identify students who might fail the course or drop out of it so that the instructors can intervene and help them. Since the classroom is in the flipped format, students have to access the reading content and attempt homework quizzes online before attending the lecture. While pedagogical methods relying on grades exist to identify such students by the middle of the semester, with the power of big data we can now find better predictive markers by leveraging not only their grades but also their clickstream data, ePortfolio, and homework submissions.
Predicting Student Dropout in MOOCs
In an effort to bring education to people without access to a teacher or the time to attend formal classes, Massive Open Online Courses (MOOCs) are being offered by universities on various MOOC platforms like edX. However, the problem of student attrition in MOOCs is very persistent across MOOCs with 90% or more students who end up dropping out of the course. In order to understand this phenomenon, we use machine learning techniques to study the behavior of students in discussion forums, patterns in video clickstreams, their performance in homework assignments and exams, as well as the emotions of students through surveys.
This research has been generously supported by the National Science Foundation, National Institutes of Health, Defense Advanced Research Projects Agency, U.S. Army Research Laboratory, U.S. Department of Energy, U.S. Department of Justice, National Institute of Justice, U.S. Department of Defense, Battelle Memorial Institute, United Way Foundation, Memorial Hospital of South Bend, Indiana CTSI, Oak Ridge National Laboratory, NET Institute, and several industry partners.