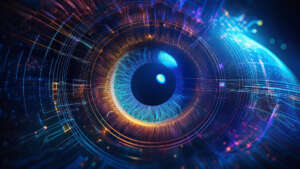
The AITAR Lab serves as a space for interdisciplinary research focused on advancing society’s trust in using biometric and AI-assisted systems that promote human flourishing at the intersection of AI, social science, and humanities.
More information about current and on-going research projects can be found below:
Human-Machine Supervision Cycle for Trustworthy Biometrics
The goal of this project is to establish a holistic framework for human-machine supervision cycle to enable (a) human-guided design of computer vision methods to make the biometric presentation attack detection mechanisms generalize better to unknown attack instruments, and (b) creation of computer-aided methods of assisting human examiners in detecting of fake inputs.
Sponsor: National Science Foundation
Trusted Artificial Intelligence and Counter Autonomy
The research goals of this project are focused around the use of human salience in unsupervised learning regime, to increase AI model generalization in tasks of interest of the NSWC Crane. This project also explores the feasibility of application of remote photoplethysmography (rPPG) sensing in assessing the mental state of human operators cooperating with the AI. As a workforce development project, its overarching goal is to train the next generation of professionals who will address the U.S. needs in the field of trusted AI.
Sponsor: US Department of Defence (via Purdue University’s SCALE program)
Partners:
- Christopher Sweet, Center for Research Computing, University of Notre Dame
- Timothy Kelley, Naval Surface Warfare Center, Crane Division
- Jessy Robins, Naval Surface Warfare Center, Crane Division
Newborn Iris Recognition
The goal of this research is to design, implement and evaluate the feasibility of iris recognition applied to newborns. The ultimate goal is to contribute to a better linkage with mothers and/or guardians, resulting in improved chances to benefit from healthcare systems, especially in developing countries.
Partners:
- Sherri Bucher, School of Medicine, Indiana University, USA
- Kathryn M. Haider, Riley Pediatric Ophthalmology, Indiana University
- Piotr Maciejewicz, Medical University of Warsaw, Poland
- Mateusz Trokielewicz, PayEye LLC, Warsaw, Poland
Sponsor: National Science Foundation
Forensic Iris Recognition
The purpose of this project is to design and deliver artificial intelligence-based biometric solutions to acquire and process images of a decedent’s iris and ocular regions for reliable automatic post-mortem identification. It extends our past NIJ-sponsored research by exploring generative AI to enhance forensic iris datasets and by utilizing saliency-guided training applied to forensic iris encoding and matching.
Partners:
- Piotr Maciejewicz, Medical University of Warsaw, Poland
- Mateusz Trokielewicz, PayEye LLC, Warsaw, Poland
Sponsor: National Institute of Justice
Visual and Audio Stimuli-Based Treatment of Mental Health
This project aims to develop a novel, non-invasive method of visual and audio stimuli-based treatment of mental dysfunctions and disorders by leveraging the similarity between modern AI models and the human brain. More specifically, our approach uses artificially-generated stimuli (pictures, videos and audio signals) in a way to stimulate selected sections of the trained artificial neural networks, which in a similar way will activate (or attenuate) parts of the human brain when these generated stimuli are perceived by humans. This interdisciplinary project brings together computer scientists, neuroscientists, psychologists, psychotherapists, and neuropsychologists to build a holistic proof-of-concept system operated on both portable devices (targeting a wider population of users) and more specialized devices using virtual reality equipment (to be used in mental health treatment facilities).
Partner: Michael Villano, Department of Psychology, University of Notre Dame
Open Source Iris Recognition
There are a plethora of commercial and academic implementations of automated algorithms for iris identification, which differ in accuracy, time of execution, and generalization capabilities (to new sensors, subjects, ethnic groups, time between enrollment and verification, etc.). However, the many NIST IREX evaluations have been populated largely by commercial (closed-source) algorithm submissions. This project leverages the capabilities of the IREX program to also incorporate open-source solutions in such evaluations for the following reasons:
- Reproducible, trustworthy, and professionally-tested algorithms would serve as an important baseline and benchmark for academic efforts to design new iris recognition methods,
- Having an algorithm from an academic unit submitted to and vetted by IREX may decrease the reluctance of the academic community to have their methods evaluated in the IREX program,
- Freely accessible, well-documented, and IREX-tested software packages may facilitate fast deployments of iris recognition in smaller-scale or pilot implementations before the adoption of professional solutions.
Outcomes of this project are available via the Open Source Iris repository.
Partner: Patrick Flynn, Department of Computer Science and Engineering, University of Notre Dame
Sponsor: National Institute of Standards and Technology (NIST)
Privacy-Safe, Identity-Preserving Biometric Data Synthesis
This project focuses on building a framework for a privacy-safe iris presentation attack detection (PAD) method, designed solely with synthetically-generated, identity-leakage-free iris images. Once trained, the method is capable of solving the PAD task in real scenarios, in which physical artifacts presented to a biometric sensor are utilized, although never being exposed to such physical artifacts during training.